Pioneering AI in MedTech Projects with Large Language Models
.png)
The fusion of Artificial Intelligence (AI) and the MedTech industry is paving the way for innovative solutions that can redefine healthcare. One of the most significant advancements within AI has been the development and deployment of Large Language Models (LLMs) like OpenAI's GPT series. These models are transforming various sectors, including MedTech. Let’s delve deeper into the systematic process of integrating AI, particularly LLMs, into MedTech projects.
1. Problem Definition and Scope:
Identifying the clinical or operational challenge is the starting point. In the context of LLMs, this could mean improving patient communication, assisting with medical literature views, or aiding in diagnostic descriptions. Mapping out the specific role of the LLM in the braoder project is essential.
2. Data Collection and Preprocessing:
- Sourcing Data: For LLMs to be effective in MedTech, they must be trained or fine-tuned on relevant medical data, ensuring domain-specific expertise.
- Preprocessing: Given the text-heavy nature of LLMs, it's crucial to structure, clean, and curate the data they're exposed to, ensuring accuracy in medical contexts.
3. Model Selection and Development:
- Choosing the Right Algorithm: LLMs excel at natural language processing tasks, making them ideal for tasks that involve text generation, comprehension, or translation in the MedTech space.
- Fine-tuning: LLMs, while pre-trained on vast amounts of general data, often benefit from fine-tuning on specific medical datasets to ensure domain relevance.
4. Validation and Testing:
- Internal Validation: LLMs must be tested rigorously on medical data, ensuring their generated content is accurate and clinically relevant.
- External Validation: Real-world testing helps gauge how well the model interacts with healthcare professionals and patients.
5. Regulatory Compliance and Ethics:
- Data Sensitivity: LLMs must respect patient confidentiality, especially when processing or generating patient-related data.
- Bias Mitigation: Ensuring LLMs do not perpetuate biases present in medical literature or data is of paramount importance.
6. Deployment and Monitoring:
- Integration: In a MedTech context, LLMs might be integrated into electronic health record systems, diagnostic tools, or patient portals.
- Feedback Loops: Constantly monitoring the outputs of LLMs ensures they remain reliable and beneficial.
7. Feedback and Iteration:
With LLMs, iterative feedback from medical professionals ensures that the generated content remains accurate, relevant, and useful.
8. Post-implementation Training and Support:
Training staff to interact with and leverage LLMs effectively ensures that the full potential of these models is realized within a medical setting.
Where AI meets MedTech, the language of innovation writes the prescription for tomorrow's healthcare - Dominik Renner
Real-world Showcases: Essentio’s Collaborative Success
Essentio, leading the way in MedTech advancements, partnered with 123Sonography. Together, we developed a state-of-the-art virtual assistant powered by AI. This tool aids users in easily locating desired content, whether videos, text, or graphics, illustrating the convergence of LLMs and MedTech.
.png)
Conclusion:
The union of AI and MedTech, particularly with the inclusion of Large Language Models, opens up new horizons for healthcare innovations. By adhering to best practices, and through fruitful collaborations like with 123Sonography, the MedTech industry stands poised to offer unparalleled benefits to both healthcare practitioners and patients.
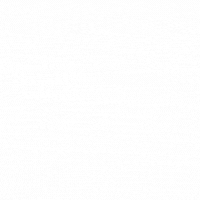